Statistical Significance for Marketers
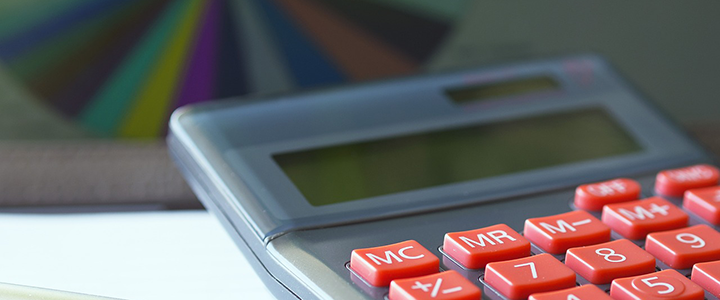
Direct marketers are all about establishing a “control” creative and always testing against the control. Why not just stick with the control if you know it works? Because there is always the possibility that changing one thing might see a lift in response so that the test creative can become the control in future campaigns.
The key is to have enough of a reach and enough responders to have results that are statistically significant. Achieving statistical significance means you can be confident that results would be duplicated in subsequent campaigns and that chance/random factors weren’t the reason a test won.
Marketers throw out the term statistically significant a lot, but for most marketers, it’s less about actual statistical equations and more of a buzzword that communicates that their AB or multivariate test had a clear winner. Establishing a winner of a test must go deeper than a greater number of calls on a postcard, higher delivery, open or click-through rates. Any test can help marketers make future format/channel/art direction decisions, but knowing if results are statistically significant and repeatable comes down to the percentage of confidence for marketers and the P-Value for statisticians or business analysts. There are some handy statistical significance calculators you could use to help you.
What audience size do I need to get a statistically significant and clear test winner?
This is the most frequently asked question by marketers. Calculators can help you go from units>conversions>conversion rate but provide little to no options to plug in a conversion number and rate to get to how many pieces/impressions to send to get clear results.
For statistical significance, we recommend 200 total responders and work backward with a good/better/best response rate. We previously posted some budgeting examples that used good/better/best response rates for different audience types (lead generation, lead nurture or current customer), and you can use those to run your own calculations.
For example, to get 200 total responders at a .25% response rate, you would need 80,000 lead gen targets to start with for a test. You can break up the 80,000 into an A and B panel and go from there. If your average response rates from your past lead gen campaigns are more/less than .25%, then use your number to calculate what audience size you would need to get 200 responders.
You could get a good read (you might not need something statistically significant) on a test using the 100 response rule. In this case, you would only need 40,000 lead gen targets to split into your test/control panels at a .25% response rate.
In any test, we only recommend changing one thing between the control and the test like the offer, color palette, layout or format. Otherwise, you won’t know which factor was responsible for the lift in response. The biggest mistake marketers make is trying to test multiple variants, which is only advisable when there are millions of outgoing mail pieces or impressions to measure.